FSOL - a workflow for the detection of patient subgroups and affected molecular features in high-throughput omics data
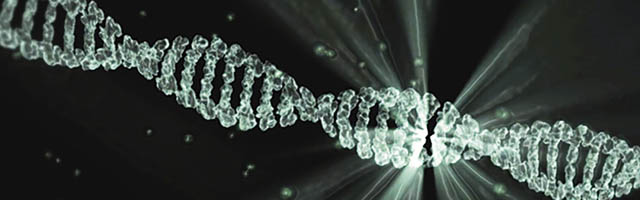
Author and article information
Abstract
In personalized medicine, one major goal is the identification of yet unknown patient subgroups with specific gene or protein expression. Different subgroups can indicate different molecular subtypes of a disease. These subtypes might correlate with disease progression, prognosis or therapy response, and the subgroup-specific genes or proteins are potential drug targets. Using high-throughput molecular data, the aim is to characterize the patient subgroup by identifying both the set of samples that shows a distinct expression pattern as well as the set of features that are affected. We present the new workflow FSOL for the identification of patient subgroups from two sample comparisons (e.g. healthy vs. diseased). First, a pre-filtering based on the univariate score FisherSum (FS) is applied to assess subgroup-specific expression of the features. FS has been shown to outperform competing methods in several settings. Second, the selected features are compared regarding the samples that form the affected subgroup. This step uses the OrderedList (OL) method that was originally developed for the comparison of result lists from gene expression studies. We compare our workflow FSOL to a reference workflow based on biclustering using real world and simulated data. On a leukemia data set, a true biological subgroup can be detected with higher stability by FSOL. On simulated data, FSOL shows higher sensitivity and accuracy compared to biclustering especially for small to moderate differences. The exploratory approach FSOL may help in identifying yet unknown mechanisms in pathologic processes and may assist in the generation of new research hypotheses.
Cite this as
2015. FSOL - a workflow for the detection of patient subgroups and affected molecular features in high-throughput omics data. PeerJ PrePrints 3:e1305v1 https://doi.org/10.7287/peerj.preprints.1305v1Author comment
The two last authors, Martin Eisenacher and Jörg Rahnenführer, contributed equally. This work has been presented at the German Conference on Bioinformatics 2015.
Sections
Additional Information
Competing Interests
The authors declare that they have no competing interests.
Author Contributions
Maike Ahrens analyzed the data, wrote the paper, prepared figures and/or tables, reviewed drafts of the paper.
Michael Turewicz analyzed the data, wrote the paper, reviewed drafts of the paper.
Katrin Marcus reviewed drafts of the paper.
Helmut E. Meyer reviewed drafts of the paper.
Caroline May reviewed drafts of the paper.
Martin Eisenacher analyzed the data, wrote the paper, reviewed drafts of the paper.
Jörg Rahnenführer analyzed the data, wrote the paper, reviewed drafts of the paper.
Funding
This work was funded by P.U.R.E., a project of Nordrhein-Westfalen, a federal state of Germany, the BMBF project de.NBI, FKZ 031 A 534A, and the Collaborative Research Center SFB 876 Providing Information by Resource-Constrained Analysis, project A3. All responsibilty for the content of this publication is assumed by the authors. The funders had no role in study design, data collection and analysis, decision to publish, or preparation of the manuscript.