Fast and accurate estimation of the covariance between pairwise maximum likelihood distances
A peer-reviewed article of this Preprint also exists.
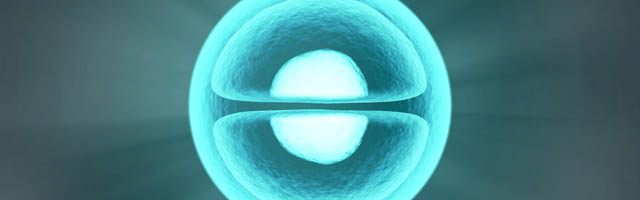
Author and article information
Abstract
Pairwise evolutionary distances are a model-based summary statistic for a set of molecular sequences. They represent the leaf-to-leaf path lengths of the underlying phylogenetic tree. Estimates of pairwise distances with overlapping paths covary because of shared mutation events. It is desirable to take these covariance structure into account in any process that compares or combines distances to increase precision. In this paper, we present a fast estimator for the covariance of two pairwise maximum likelihood distances, estimated under general Markov models. The estimator is based on a conjecture (going back to Nei and Jin, 1989) which links the covariance to path lengths. We prove it here under a simple symmetric substitution model. In a simulation, we show that our estimator outperforms previously published ones in terms of the mean squared error.
Cite this as
2014. Fast and accurate estimation of the covariance between pairwise maximum likelihood distances. PeerJ PrePrints 2:e387v2 https://doi.org/10.7287/peerj.preprints.387v2Author comment
This submission is part of the Gaston Gonnet Festschrift.I have changed some formulations, removed typos and added the sentence: "Alternatively, a nonparametric bootstrap can be used (Efron and Tibshirani, 1993), but it takes substantially longer computation times and an requires an MSA too."
Sections
Additional Information
Competing Interests
I declare that I have no competing interests that might have influenced this manuscript.
Author Contributions
Manuel Gil conceived and designed the experiments, performed the experiments, analyzed the data, contributed reagents/materials/analysis tools, wrote the paper, prepared figures and/or tables, reviewed drafts of the paper.
Funding
This work was supported by the Swiss National Science Foundation (SNF) grant PBEZP2_140129. The funders had no role in study design, data collection and analysis, decision to publish, or preparation of the manuscript.